What I’m doing is separating, with a special emphasis on differences that are hardly there. It may be human (or is it just me?) nature to be preoccupied with discrimination.
Quantitative prediction formulae to predict the skin irritancy of cosmetic ingredients are not accurate. The reason for this was taught to students using the Self Organisation Map (SOM).
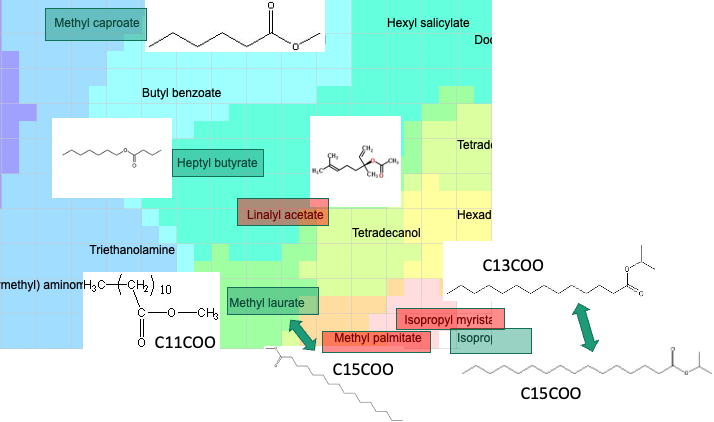
If you look closely at the clumped ester compounds, you will see some compounds circled in red, which are skin irritants, and others circled in green, which are not irritants.
The same methyl ester can be irritating only if the length of the carboxylic acid is increased.
But with isopropyl esters, on the other hand, if the carboxylic acid is smaller, it becomes irritating.
Differences that can rationally explain this difference have to be found and separated.
That’s because if you deep learn, the neural network will find some minor differences and discriminate. It would be a black box inside.
But it’s not always the molecules that are responsible.
In other countries, when it became illegal to discriminate on the basis of skin colour or gender, they started discriminating on the basis of fatness or smoking. Skin colour and gender cannot be changed, but being fat is up to the individual’s own efforts, and smoking is up to the strength of will, so it is his/her responsibility.
If you discriminate against the skin as being foreign because it is not the responsibility of the molecule itself, but because it is not very common in nature and humans have not had the chance to come into contact with it in the first place, then it cannot fit in with any molecular descriptor you bring.
I will, at best, enjoy discriminating between molecules, materials, ingredients and medicines.